AI Chatbot Success: Picking the Perfect Framework
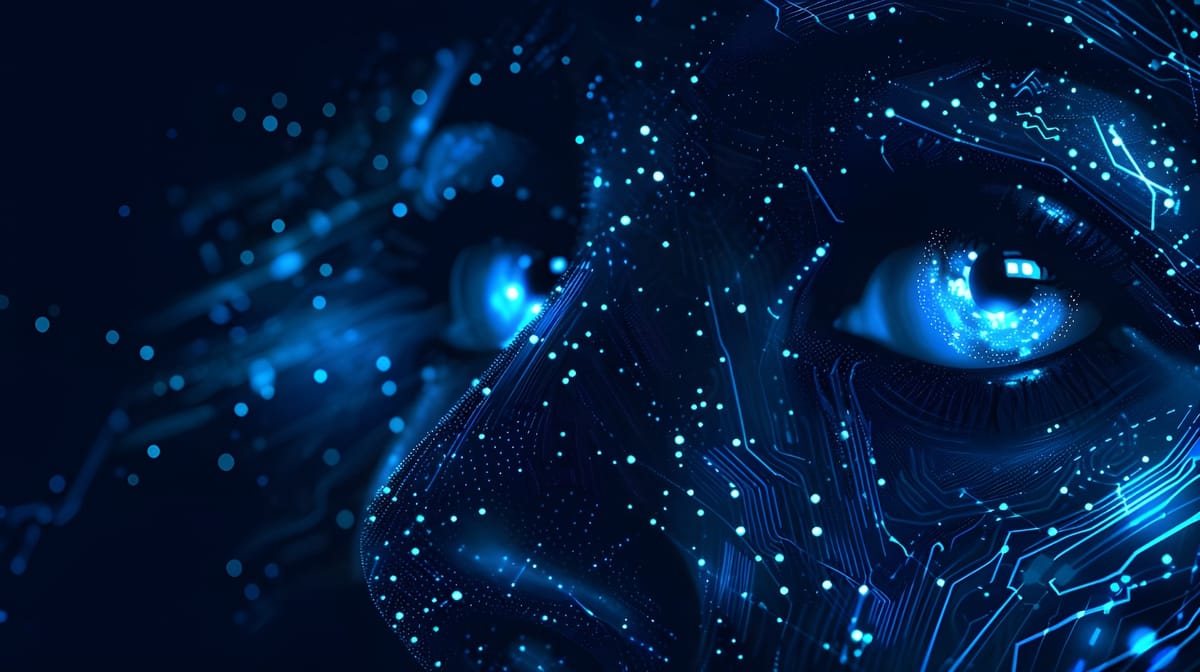
In the dynamic world of artificial intelligence, chatbots have emerged as revolutionary tools, transforming how businesses engage with customers. Selecting the right AI framework for creating these chatbots is crucial, as it can significantly impact the effectiveness and efficiency of your customer service operations. This article delves into evaluating top AI frameworks and shares insights on implementing your choice effectively, ensuring you harness the full potential of AI chatbots to enhance your customer relations.
Evaluating Top AI Frameworks
When venturing into the realm of AI chatbots, the first step is to evaluate available frameworks. A top contender is TensorFlow, developed by Google, well-known for its robust, flexible features that cater to both beginners and advanced users. It supports multiple languages and has a vast ecosystem, making it ideal for developing sophisticated chatbot systems. Another framework worth considering is PyTorch, favored for its ease of use and dynamic computation graph that allows for adjustments as you go, which is particularly beneficial during the development phase.
Choosing the right framework also depends on the specific needs and technical capabilities of your organization. For instance, Microsoft’s Bot Framework is excellent for enterprises looking for integration with Microsoft products and services. Meanwhile, Rasa shines for those prioritizing open-source solutions with strong community support. When evaluating these frameworks, consider factors like scalability, ease of integration, support for multiple languages, and the learning curve for your team.
Lastly, it’s essential to test these frameworks against real-world scenarios that your AI chatbot is expected to handle. This includes understanding the framework’s capabilities in natural language processing, machine learning efficiency, and multi-turn conversation handling. Tools like Dialogflow offer robust analytics and testing features that can help fine-tune the chatbot before full-scale deployment. Ensuring the framework can handle your specific requirements will save significant time and resources in the long run.
Implementing Your Choice Effectively
Once you have selected an AI chatbot framework, effective implementation is key to unlocking its potential. Begin by setting clear objectives for what the chatbot must achieve — whether it’s handling customer inquiries, supporting sales, or providing personalized recommendations. These goals will guide the customization of the chatbot to address specific business needs. Additionally, involve stakeholders from various departments early in the process to align the chatbot’s functionality with broader business objectives.
Training the chatbot with high-quality data is crucial for its performance. This involves not only programming it with relevant FAQs and responses but also continually updating it with new insights gathered from actual interactions. Utilize the machine learning capabilities of your chosen framework to refine the chatbot’s responses and improve its interaction quality over time. For example, TensorFlow provides tools that can help automate and streamline this training process, making the chatbot smarter and more efficient as it learns from its interactions.
Finally, ensure robust integration of the chatbot into your existing digital infrastructure. Seamless integration into websites, mobile apps, and customer relationship management (CRM) systems enhances user experience and accessibility, making it easier for customers to interact with the chatbot. Regularly review performance metrics and user feedback to iterate and evolve the chatbot’s capabilities. This ongoing optimization will help maintain a high level of customer satisfaction and operational efficiency.
Choosing and implementing the right AI chatbot framework is not just about leveraging technology but about transforming how businesses interact with their customers in a digital-first world. By meticulously evaluating AI frameworks and focusing on effective implementation, companies can ensure that their chatbot initiatives drive customer engagement and operational efficiencies. Remember, the journey doesn’t end with deployment; it’s an ongoing process of learning and adaptation to continually meet and exceed customer expectations.