Machine Learning's Impact on Autonomous Support Systems
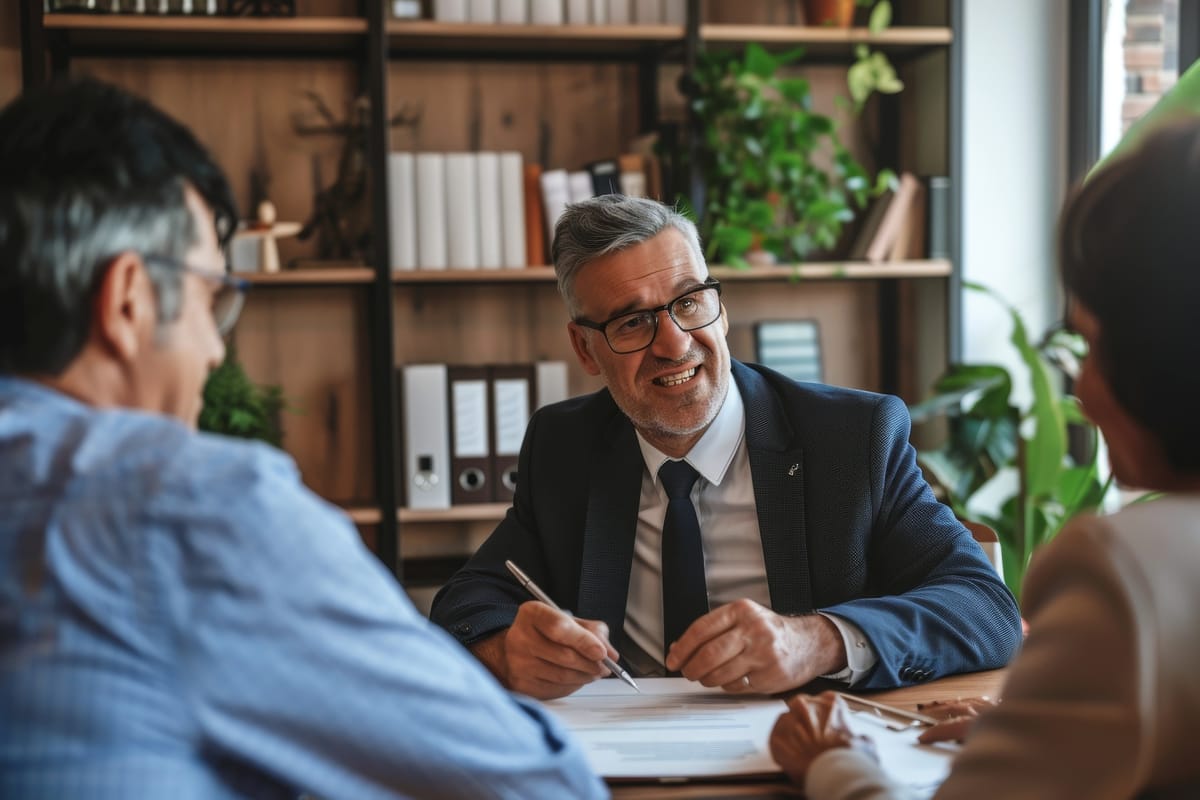
The Revolution of Machine Learning
Machine learning has revolutionized various industries, and one area where its impact is particularly profound is in the development of autonomous support systems. These systems, which rely on artificial intelligence (AI) and machine learning algorithms, have the potential to greatly enhance efficiency, decision-making, and safety in a wide range of applications. From self-driving cars to industrial robots, the integration of machine learning has opened up new possibilities for autonomous systems. In this article, we will explore the evolution of machine learning in autonomous support systems and discuss its impact on efficiency, decision-making, predictive maintenance, safety, and reliability.
Autonomous Support Systems: An Overview
Autonomous support systems refer to technologies that can perform tasks and make decisions without human intervention. These systems are designed to operate independently, using sensors, actuators, and advanced algorithms to navigate and interact with their environment. Examples of autonomous support systems include self-driving vehicles, robotic process automation (RPA) systems, and intelligent industrial machines.
The key advantage of autonomous support systems is their ability to operate in complex and dynamic environments, where human intervention may be impractical or unsafe. These systems can analyze vast amounts of data in real-time, learn from their experiences, and adapt their behavior accordingly. This level of autonomy allows them to perform tasks more efficiently, accurately, and consistently than human operators.
The Evolution of Machine Learning in Autonomous Support Systems
Machine learning has played a crucial role in the evolution of autonomous support systems. In the early days, these systems relied on rule-based algorithms that were programmed to perform specific tasks. However, these algorithms had limited flexibility and struggled to adapt to changing conditions.
With the advent of machine learning techniques, autonomous support systems became capable of learning from experience and improving their performance over time. By analyzing large datasets, machine learning algorithms can identify patterns, make predictions, and optimize their decision-making processes. This enables autonomous systems to handle complex and unpredictable situations with greater efficiency and accuracy.
Enhancing Efficiency: Machine Learning’s Impact on Autonomy
Machine learning has significantly enhanced the efficiency of autonomous support systems. By analyzing vast amounts of data, these systems can identify patterns and optimize their operations to achieve better performance. For example, in self-driving cars, machine learning algorithms can analyze sensor data to improve navigation, reduce fuel consumption, and minimize traffic congestion.
In industrial settings, machine learning algorithms can optimize production processes by analyzing sensor data and identifying areas for improvement. These algorithms can detect anomalies, predict equipment failures, and optimize maintenance schedules, leading to increased productivity and reduced downtime.
Advancements in Decision-Making with Machine Learning
One of the key benefits of integrating machine learning into autonomous support systems is the advancement in decision-making capabilities. Traditional rule-based algorithms often struggled to handle complex and unpredictable situations, as they relied on predefined rules and lacked the ability to adapt.
Machine learning algorithms, on the other hand, can learn from vast amounts of data and make informed decisions based on patterns and trends. This enables autonomous support systems to handle dynamic and uncertain environments more effectively. For example, in autonomous drones, machine learning algorithms can analyze sensor data to make real-time decisions about obstacle avoidance, route planning, and object recognition.
Machine Learning’s Role in Predictive Maintenance
Predictive maintenance is another area where machine learning has had a significant impact on autonomous support systems. By analyzing sensor data and historical maintenance records, machine learning algorithms can predict equipment failures before they occur. This allows for proactive maintenance, reducing downtime and improving overall system reliability.
In industries such as manufacturing and transportation, predictive maintenance can save millions of dollars by avoiding unplanned downtime and minimizing the risk of equipment failure. Machine learning algorithms can identify patterns and anomalies in sensor data, allowing maintenance teams to take timely action and prevent costly breakdowns.
Improving Safety and Reliability through Machine Learning
Machine learning also plays a crucial role in improving the safety and reliability of autonomous support systems. By analyzing sensor data and learning from past experiences, machine learning algorithms can identify potential risks and take proactive measures to mitigate them.
In self-driving cars, for example, machine learning algorithms can analyze sensor data to detect and classify objects on the road, such as pedestrians, cyclists, and other vehicles. This enables the autonomous system to make informed decisions and take appropriate actions to avoid accidents.
Similarly, in industrial settings, machine learning algorithms can analyze sensor data to detect anomalies and potential safety hazards. By identifying these risks in real-time, autonomous support systems can take immediate action to prevent accidents and ensure the safety of operators and equipment.
Challenges and Limitations in Implementing Machine Learning
While machine learning offers significant benefits to autonomous support systems, there are also challenges and limitations that need to be addressed. One of the main challenges is the availability of high-quality and labeled training data. Machine learning algorithms rely on large datasets to learn and make accurate predictions. However, obtaining and labeling such datasets can be time-consuming and expensive.
Another challenge is the interpretability of machine learning models. In some cases, machine learning algorithms make decisions based on complex patterns that are difficult to interpret by humans. This lack of interpretability can raise concerns, especially in safety-critical applications where human oversight is necessary.
Furthermore, machine learning algorithms require significant computational resources to process and analyze large amounts of data. Implementing these algorithms in resource-constrained environments, such as embedded systems or edge devices, can be challenging.
The Future of Autonomous Support Systems with Machine Learning
The future of autonomous support systems looks promising with the continued integration of machine learning. As machine learning algorithms become more sophisticated and capable, autonomous systems will be able to handle increasingly complex tasks and operate in more challenging environments.
Advancements in machine learning techniques, such as deep learning and reinforcement learning, will further enhance the decision-making capabilities of autonomous support systems. These algorithms will enable autonomous systems to learn from unstructured data, make more accurate predictions, and adapt their behavior in real-time.
With the increasing availability of big data and advancements in computing power, machine learning algorithms will have access to larger and more diverse datasets. This will enable autonomous support systems to learn from a wider range of scenarios and improve their performance across different domains.
Embracing the Potential of Machine Learning
Machine learning has revolutionized the field of autonomous support systems, enabling them to operate more efficiently, make better decisions, and improve safety and reliability. As technology continues to advance, the integration of machine learning will only become more prevalent, leading to further advancements in autonomous systems.
By leveraging the power of machine learning, autonomous support systems have the potential to transform industries and improve our daily lives. From self-driving cars that make our commutes safer and more efficient to intelligent robots that optimize industrial processes, the impact of machine learning on autonomous support systems is undeniable.
As we embrace the potential of machine learning, it is important to address the challenges and limitations associated with its implementation. By addressing these issues, we can ensure the responsible and effective integration of machine learning into autonomous support systems, unlocking their full potential and reaping the benefits they offer.